Project Description
Urbia: Urban Insights Atlas. Using the Digital Atlas of Australia (map, api, and data), and other relevant local data, UrbIA allows users to explore their environments and receive AI-generated insights on road safety, capacity forecasting, and urban sustainability. With UrbIA, users can react to insights, provide suggestions, and contribute to improving their communities.
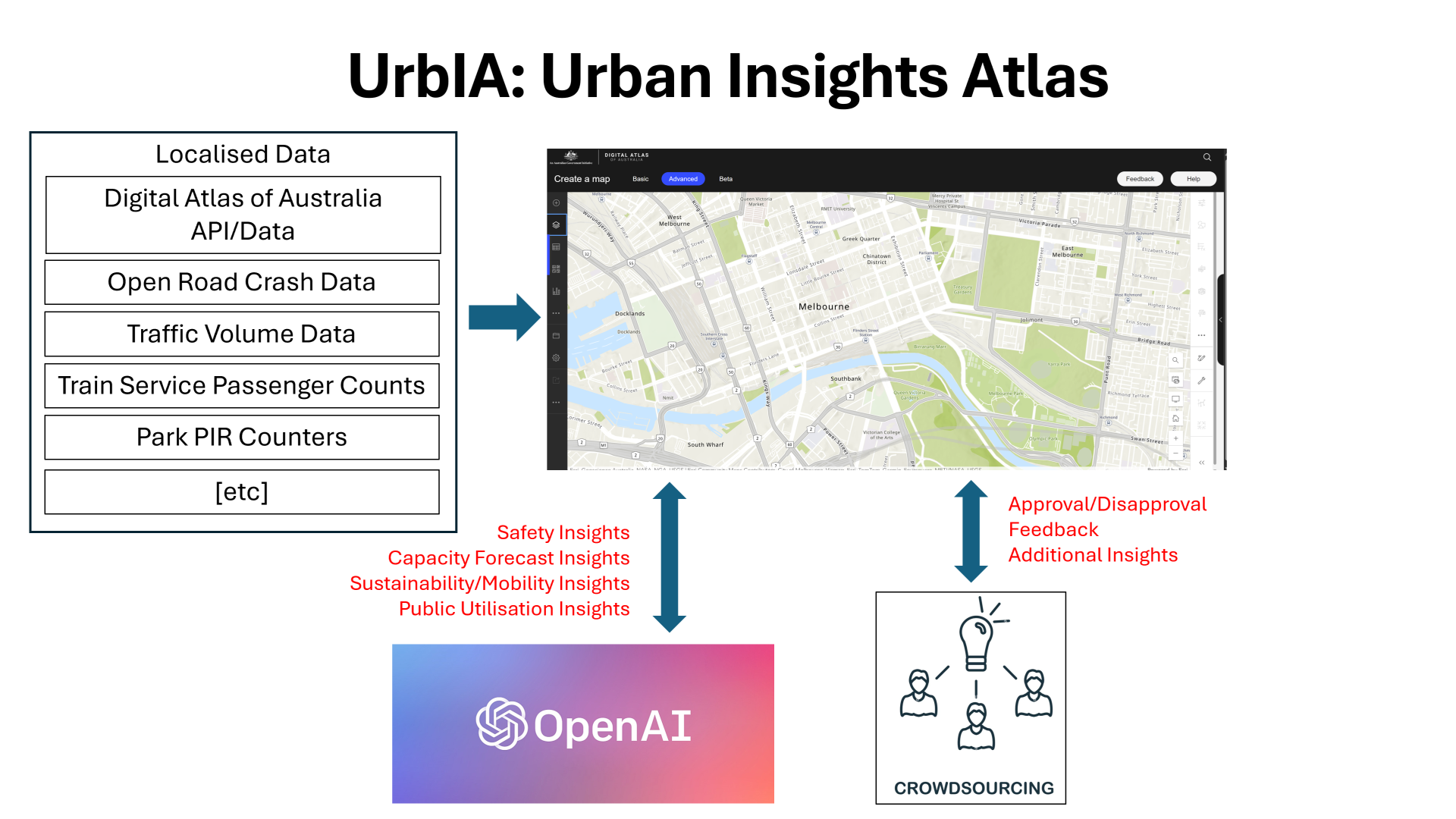
Introduction
The Banico Family proudly presents our GovHack project, UrbIA (Urban Insights Atlas), an innovative tool designed to make geospatial data accessible to everyone, empower decision-making, and promote sustainable urban development across Australia.
Features and Technical Approach
- Geospatial Navigation: Users interact with a map interface powered by the Digital Atlas of Australia, which presents localized data across categories such as road safety, sustainability, and public services.
- Integration with the Digital Atlas of Australia: UrbIA will dynamically retrieve geolocated data sets as input for insights. (https://digital.atlas.gov.au/api/search/definition/)
- Generative AI for Insights: Through OpenAI, UrbIA generates tailored insights based on real-world data. Users can rate these insights and suggest improvements, creating a feedback loop between data-driven analysis and community input.
- Crowdsourced Wisdom: By allowing users to react and provide suggestions, UrbIA encourages collective knowledge sharing, empowering both citizens and policymakers to make informed decisions.
Use Case Highlights
- Safety Insights: For road safety, UrbIA can analyze Victorian Road Crash Data and generate actionable recommendations, such as improving road lighting and adding guardrails.
- Capacity Forecasting: Using traffic volume data, UrbIA can assess roads for capacity needs, recommending maintenance and safety improvements where necessary.
- Sustainability Insights: UrbIA examined cycling infrastructure and public transport utilization to propose strategies for reducing car dependency and promoting green mobility.
Meeting the Challenges
UrbIA addresses several critical questions:
1. How can we use the Digital Atlas of Australia’s API and Generative AI to create user-friendly tools and visualizations that make geospatial data accessible, empowering decision-making and engagement with local and national environments?
Our solution leverages the Digital Atlas of Australia, geolocated data, and AI to offer users an intuitive map-based platform. Users can explore local data (e.g., road safety statistics, traffic patterns, housing demand) while receiving AI-generated insights. These insights guide decision-making by offering actionable suggestions, like improving infrastructure or enhancing road safety.
2. How might we leverage road crash statistics and multi-agent AI-based applications to enhance road safety and inform policy-making?
For road safety, UrbIA integrates Victorian Road Crash Data to pinpoint accident locations, types, and times. It leverages AI to generate recommendations such as improved road lighting, safety barriers, or targeted public awareness campaigns. Users can contribute to these insights by providing feedback, fostering a collaborative approach to road safety enhancements.
3. How might we predict future changes in community dynamics, such as population density, housing demand, traffic patterns, and the demand for public services or amenities?
While UrbIA primarily focuses on current geospatial data, it uses AI to generate capacity insights based on traffic patterns and public service utilization. By analyzing geolocated data, such as road traffic volumes or public transport usage, UrbIA provides AI-driven recommendations on infrastructure capacity. For example, it can suggest where roads might need upgrades or where public services could be expanded based on current usage patterns. These AI-generated insights allow councils and policymakers to make informed decisions to address immediate community needs and optimize urban planning efforts.
4. How can we use data insights to promote the development of sustainable urban infrastructure and reduce dependency on private vehicles?
UrbIA analyzes geolocated data on bike paths, public transport usage, and traffic patterns, using AI to generate insights that promote sustainable alternatives. For example, the AI can suggest areas where cycling infrastructure could be expanded or where public transport services might be optimized based on current usage patterns. These AI-driven recommendations help urban planners focus on reducing car dependency and encourage greener mobility solutions, fostering the development of more sustainable urban environments.
5. How can councils leverage climate and movement data from multi-function poles, sensors, and devices to improve asset management, optimize services, and design cleaner, more livable urban spaces?
UrbIA integrates climate and movement data from sensor-equipped urban assets and uses AI to generate insights for better asset management. For example, AI can suggest adjustments to street lighting based on movement patterns or identify areas that would benefit from green infrastructure to mitigate urban heat island effects. These AI-generated recommendations allow councils to optimize services, improve urban livability, and make data-driven decisions that support cleaner, more efficient public spaces.
Sample Insights Generated by UrbIA
Please check Github our repository for the actual generated documents: https://github.com/jasonabanico/UrbIA/tree/main/src/Output%20Data
Safety Insights Suggestions (generated from Victorian Road Crash Data)
- Improve road lighting in rural and high-speed zones, especially at known accident hotspots, to enhance visibility and reduce the risk of collisions with fixed objects.
- Implement additional safety measures such as guardrails and better signage to prevent vehicles from running off the road, particularly in dark, undivided arterial roads.
- Conduct targeted awareness campaigns and driver education programs focusing on the dangers of driving at high speeds on rural roads, especially for young drivers who are statistically more likely to be involved in such incidents.
Capacity Forecast Insights Suggestions (generated from Traffic Volume Data)
- Considering the low Average Annual Daily Traffic (AADT) and truck percentage, there may not be an immediate need for significant capacity upgrades on Marysville-Woods Point Road.
- With the observed growth rate of 1.3%, periodic monitoring and minor improvements could suffice to handle future traffic increases without major infrastructure changes.
- Given the road's classification as "C Class" and "Arterial Other," focus on maintaining road quality and safety measures, particularly around identified peak times and specific segments with higher traffic density.
Sustainability/Mobility Insights Suggestions (generated from Principal Bicycle Network Proposed Paths)
- Promote Sustainable Transport: Advocate for the rapid development and implementation of the proposed off-road cycling route at South Wharf to encourage sustainable and eco-friendly transportation, reducing reliance on motor vehicles and lowering carbon emissions.
- Enhance Infrastructure for Safety and Comfort: Ensure the proposed promenade includes high-quality facilities such as adequate lighting, smooth surfaces, and sufficient width to accommodate all users safely, improving the overall experience and encouraging more people to cycle.
- Community Engagement and Feedback: Engage with the local community for feedback on the proposed cycling route to ensure it meets the needs of residents and commuters, fostering a sense of ownership and increasing the likelihood of its successful adoption and use.
Sustainability/Mobility Insights Suggestions (generated from Train Passenger Service Count Data)
- The data indicates low passenger activity at Sunbury station for early morning Metro services on a normal weekday, suggesting an opportunity to optimize train schedules and reduce energy consumption by running fewer trains during these low-demand periods.
- Implementing demand-responsive scheduling and potentially smaller, more energy-efficient trains or carriages during off-peak hours can reduce operational costs and carbon footprint.
- Encouraging public transportation usage through targeted campaigns or incentives during these times may help balance train loads and improve the overall sustainability of the service.
Public Utilisation Insights Suggestions (generated from Scenic Reserve PIR Counter)
[Developer's Note: Additional Data from other sources may be required to improve suggestion quality, as the output below is quite specific to the counter.]
- The anomalously high
peopleCount
of 500 on 2023-09-26 at 01:00:00 suggests a possible error or unusual event that should be investigated for data accuracy.
- The consistent battery levels around 3.62 to 3.64 volts indicate stable device performance, but regular monitoring is recommended to ensure uninterrupted data collection.
- The
nonNegPeopleCount
often matching the peopleCount
except for specific instances like 2023-09-27 and 2023-10-05 suggests that some readings may require validation to ensure accurate visitor tracking at Mary Cairncross Scenic Reserve.
Conclusion
Our project demonstrates how geospatial data and AI can transform decision-making in urban planning, road safety, and sustainability. With UrbIA, we empower all Australians to engage with their environments and help shape the future of their cities.
Data Story
UrbIA combines geolocated open data from the Digital Atlas of Australia (as well as other sources), OpenAI, and crowdsourced feedback, to generate insights to aid in urban planning and development.
Data Flow: Geolocated open data (geojson) / Digital Atlas of Australia > UrbIA + OpenAI > User Rating and Feedback
UrbIA aids in urban planning by utilizing a diverse array of geospatial data to enhance areas such as safety, capacity planning, sustainability, and service use. The platform integrates information from the Digital Atlas of Australia and various public datasets — such as road crash statistics, traffic volume reports, proposed bicycle paths, train station passenger counts, and public service utilization.
Generative AI, powered by OpenAI, processes this data to generate tailored recommendations, such as infrastructure improvements based on identified accident hotspots or traffic patterns. The key to the generated output is using keywords such as "safety insights", "capacity forceast insights", and other similar insights, when doing prompt engineering.
Users refine these insights through rating and feedback, creating a collaborative loop that enhances the relevance and accuracy of the recommendations.
Users interact with an intuitive map interface, allowing them to explore localized data across multiple categories and gain actionable insights for improving their locality.
UrbIA’s practical use cases include analyzing Victorian Road Crash Data to suggest safety enhancements, assessing traffic volume data for capacity forecasting and maintenance needs, and examining cycling infrastructure and public transport usage to promote green mobility solutions.
By incorporating crowdsourced wisdom, UrbIA empowers both citizens and policymakers with actionable, data-driven insights, fostering a more informed and engaged community in the development of sustainable urban environments.